Our personal data is a highly valuable commodity to a variety of businesses and agencies. This information is collected for a variety of reasons, and while some of the data can be fairly generic, other information—like surnames, birth dates, social security numbers, and account numbers—is more intimately linked with a person’s identity. In the wrong hands, this data can be exploited for nefarious purposes. As a result, there is a need to take measures to anonymize this personal data and minimize any consequences of a potential data breach or the risk of re-identification of individuals in published work. But what exactly do we mean by data anonymization?
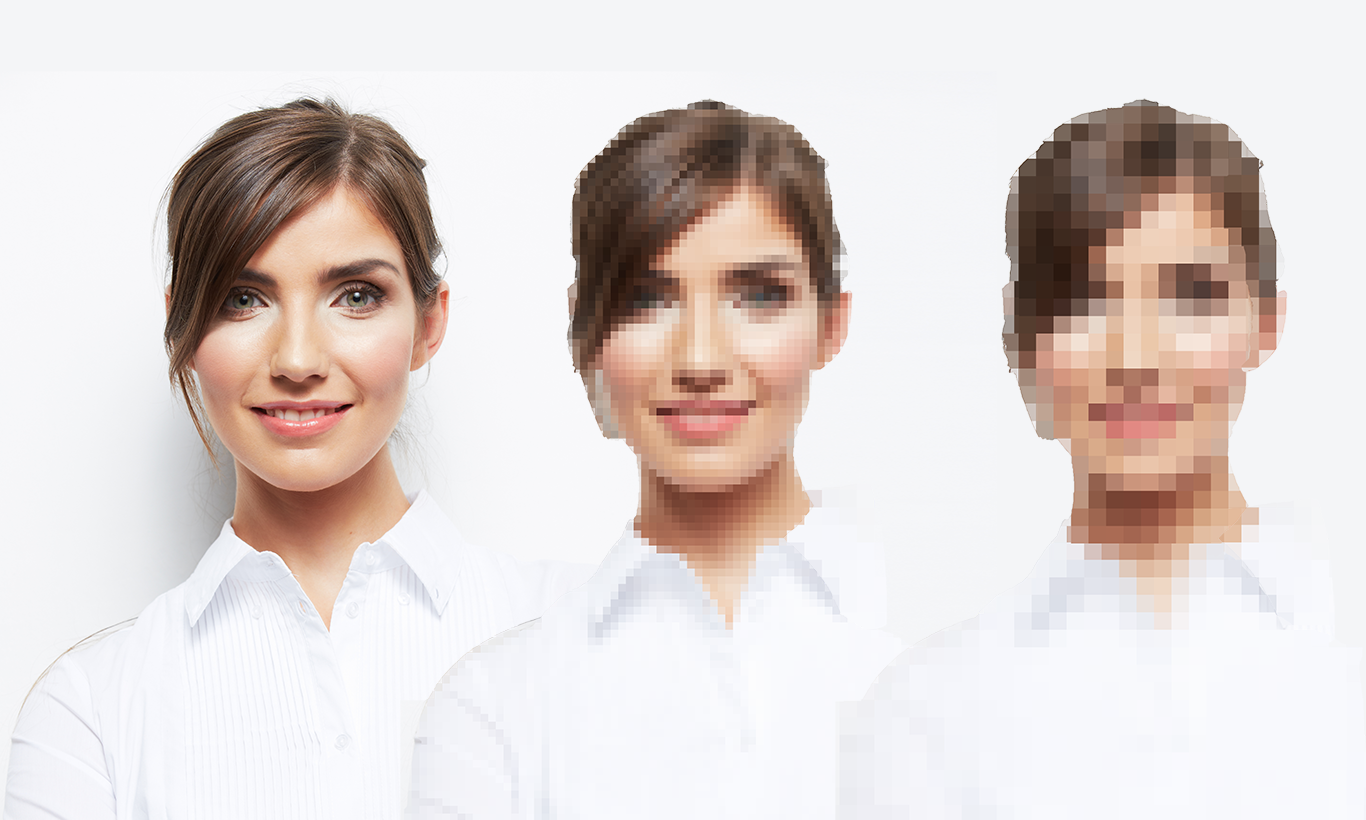
What is Data Anonymization?
Data anonymization, also known as data redaction, is the process of removing or concealing the identifiable information of individuals (i.e., personal data), so that the data may be used more widely in different applications. Several organizations, such as the Institutional Review Board (IRB) and European Medicines Agency (EMA), require researchers and companies to anonymize their data before sharing or publishing their work, in order to protect the privacy of their data subjects and their personal data.
Article 3(1) in the Regulation (EU) 2018/1725 of the European Parliament defines personal data and data subjects as follows:
[P]ersonal data’ means any information relating to an identified or identifiable natural person (‘data subject’); an identifiable natural person is one who can be identified, directly or indirectly, in particular by reference to an identifier such as a name, an identification number, location data, an online identifier or to one or more factors specific to the physical, physiological, genetic, mental, economic, cultural or social identity of that natural person[.]”
Of course, depending on the context of the data release, the anonymization requirements may change. For example, internal data sharing would have less strict anonymization requirements as opposed to a public release of a dataset.
According to the EMA, effective anonymization solutions may be evaluated on three criteria:
- Possibility to single out an individual.
- Possibility to link records relating to an individual.
- Whether information can be inferred with regard to an individual.
If an anonymization solution fails in one of the three criteria, then the risks of re-identification must be evaluated.
What are the challenges?
There are several challenges associated with efficient data anonymization, the main one being the achievement of balance between anonymization and readability. Anonymized data is often used for training data models to predict certain characteristics, behaviors, or outcomes in a variety of fields. However, a dataset that has an important part of its data redacted may not be very useful for further analyses and may affect the performance of the models. Consequently, there is always a trade-off between privacy and model performance to consider.
With the rise of Big Data and the Semantic Web, another challenge has appeared: the risk of re-identification due to linked databases. The Semantic Web, or Web 3.0, allows linking diverse information about individuals across databases that may be used for Artificial Intelligence processes. However, this increases the difficulty of anonymizing personal data efficiently, since there are pieces of identifiable information in multiple locations. Even if we anonymize personal data in one database, we may be able to re-identify an individual based on the linked identifiable information in a different database.
Finally, there are challenges in identifying and extracting personal data properly, but also in making the anonymization reversible. Many companies use manual annotation systems that add tags to the information, or entities, that need to be anonymized. The available volume of data nowadays makes this process extremely time-consuming and labor-intensive. In addition, a good anonymization solution should also use secure encryption for saving the original information and allowing the reversal of anonymization: the de-anonymization of data. For these reasons, there is increased demand for automated systems that efficiently and accurately identify different types of personal data in unstructured text.
How is it done?
Anonymization may be approached from a utility or a privacy viewpoint. The utility approach focuses on preserving the utility of the data as much as possible and allows for some loss of privacy, whereas the privacy approach focuses on implementing methods that offer the highest privacy while sacrificing some of the data utility. Depending on the data release context, we may choose one or the other; however, an ideal anonymization solution should strike a balance between privacy and utility.
The two main steps in a data redaction task are the data preprocessing and the anonymization.
Data Preprocessing
During the data preprocessing step, we make sure that our data is formatted appropriately and as clean as possible. For example, spelling mistakes may decrease accuracy when identifying the personal information that needs to be anonymized. The identification of information, or entities, that need to be anonymized is also part of the data preprocessing step, and it is achieved either via manual tagging or an automated approach; of course, the latter is preferred. Once this information is identified and tagged, it is classified into direct identifiers and quasi-identifiers based on replicability, distinguishability, and knowability.
Anonymization
There are several considerations that we need to take into account when moving onto the anonymization step. First, we need to identify any possible attacks to our data and the entities behind them. Where will the data be released? Who will have access to it? Could it be used in a malicious way? If so, how? The answers to these questions help us evaluate and decide on the balance between low re-identification risk and data usability.
Next, we need to choose an anonymization methodology that is appropriate for our selected privacy-utility balance and data goals. The simplest anonymization technique is the complete and irreversible removal of any personal information using a data redaction software. While this offers a very low risk of re-identification, it scores very low in data utility since the de-identified data is not very readable.
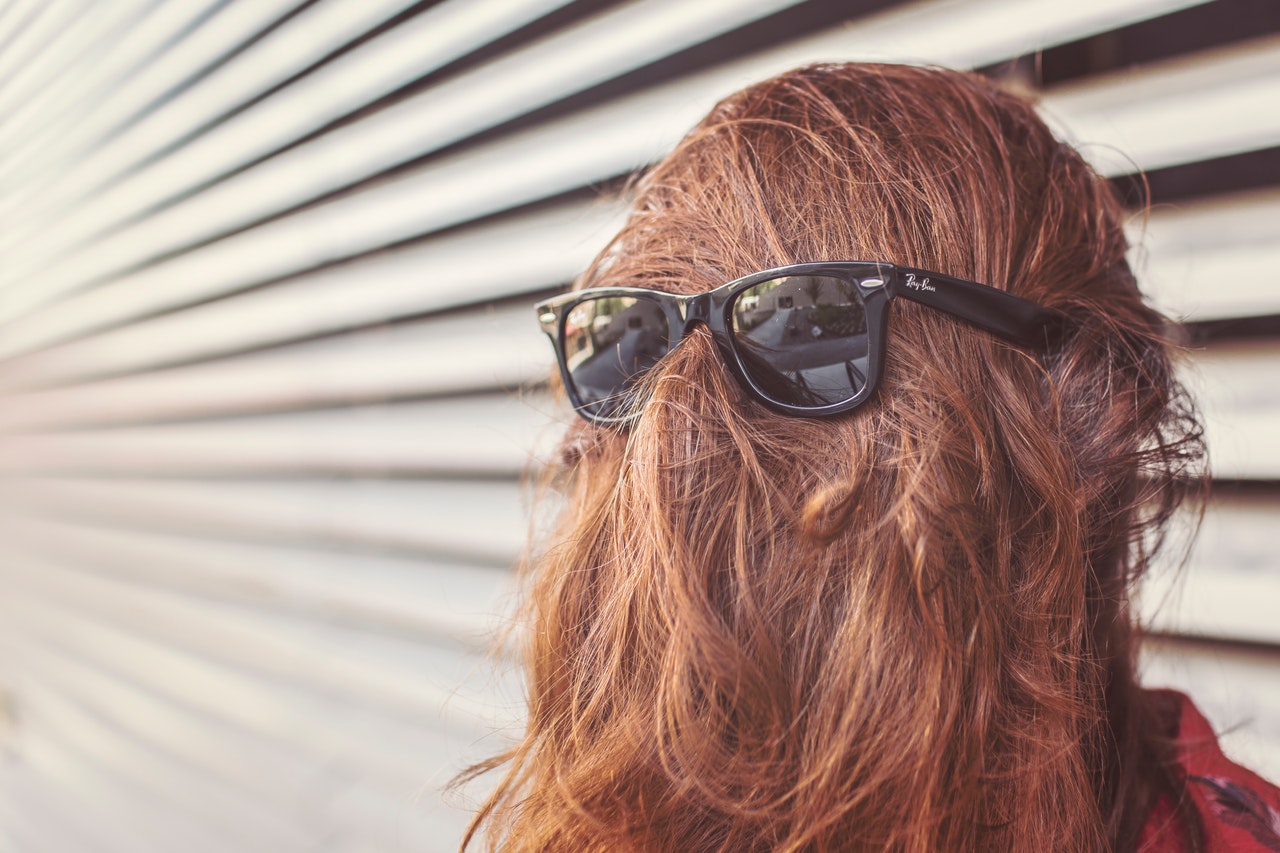
Another method is pseudonymization, where personal information is replaced with terms that indicate the type of information (such as “Redacted Name” or “Redacted Address”). Even though this method makes the text more readable, it is difficult to track specific points of interest among multiple documents for research purposes. An alternative technique is the use of unique numeric or alphanumeric sequences that allow tracking among documents; when combined with a helpful term for the type of information redacted, they also offer high readability.
While pseudonymization makes the anonymized text more readable, it also increases the risk of re-identification via quasi-identifiers: if enough non-direct pieces of personal information are combined, one may be able to infer the identity of an individual. For this reason, the use of algorithms that use a generalization or a randomization approach have been introduced into data redaction tasks in order to decrease the possibility of re-identification even when using quasi-identifiers scattered in our data. The generalization approach replaces a value with a range (e.g., age value 53 replaced with range 45-55), and k-anonymity is a popular generalization method that ensures an individual cannot be identified since they are grouped with k-1 other participants in that range. Even though k-anonymity is a great choice for structured data, it does not work well with unstructured text and results in less accuracy when creating machine learning models because of information loss. In contrast, the randomization approach (noise addition and permutation, e.g. shifting dates randomly backward or forward) may cause issues with relationships between attributes, but is more suited for unstructured text. More specifically, the method based on the differential privacy concept has recently become very popular because of its low impact on model accuracy: it is used to anonymize the data by randomizing values or adding noise so that a query cannot verify whether or not an individual is part of a database. Despite its popularity, this method is fairly complex to implement.
Documentation Required
Finally, whatever methodology we decide to use, we need to make sure that we document it thoroughly. In certain cases, the anonymization methodology documentation may be a requirement by regulatory agencies, such as the EMA, but it is also considered a good data science practice in general.
Megaputer offers an automated solution for both the data preprocessing and anonymization parts of a data redaction task. If you are interested in learning more details about how you can anonymize your data using PolyAnalyst™, feel free to contact us for a free demo.