Online communication, also known as Computer-mediated Communication (CMC), is any communication among individuals via networked devices, such as computers and mobile phones1. Online communication is not only textual, but may include other modes of communication: images, audio, video, or a combination of those. For example, we may participate in a Facebook conversation using a GIF animation along with text or we may use video, audio, and text at the same time when making a Skype call. Even though our online communication is becoming increasingly multimodal, the vast majority is still textual. As a result, we have to make sure that the text in our data is in a format comprehensible by text analysis tools.
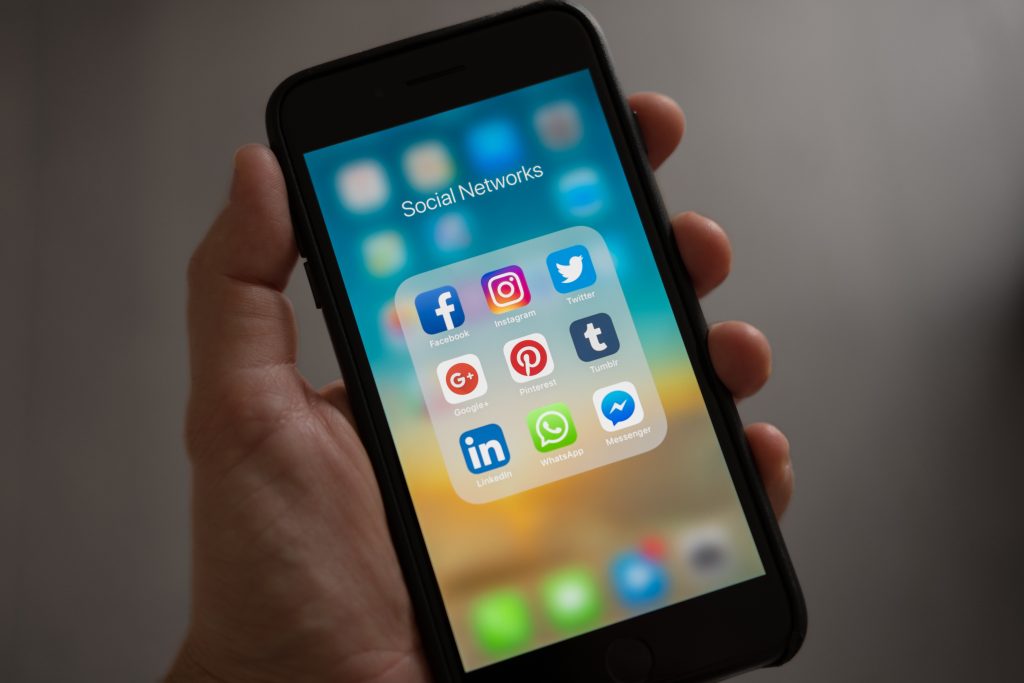
Why is understanding online communication important?
The popularization of computers, and, more recently, social media, has drawn a lot of attention to the vast amounts of user-generated content shared daily. According to recent reports2, approximately 2.5 quintillion bytes of data are created every day. All this data contains useful information that can be extracted and analyzed in order to make better business decisions. Nowadays, a number of companies rely on customer reviews and opinions about their products and services on social media, rather than old-fashioned surveys that may take a long time to distribute and acquire. Technological mechanisms such as tags or hashtags make it easier to track and collect data about specific topics that we can analyse using Sentiment Analysis, in order to understand the feelings and opinions of our customers toward our business. Moreover, we can identify and track how trends spread over different communities and locations using Social Network Analysis, or predict how new trends may evolve. However, as useful as analyzing online communication is, it comes with certain challenges.
What are the challenges of analyzing online communication?
The most common challenges and analyst mistakes with regard to online communication are associated with identifying and understanding the communicative behaviors in our data source. Those behaviors may be affected by both the technological environment and the social structure of the specific source3. There is a common misconception that all online communication is characterized by as specific set of features, commonly referred to as “netspeak”: emoticons, abbreviations, acronyms, transliteration of words with symbols and numbers, etc. However, online communication has a variety of forms just like offline communication.
We use different language when we chat with a friend than in a conversation with our boss, or when we leave a quick note on the fridge as opposed to a formal letter. Similarly, we use different language when we chat on Facebook messenger with our friend, when we write a tweet, or in an email to our boss or professor.
w8t waaaaaat U+1F928
↓
wait what [emoji: face with raised eyebrow]
Our language also varies based on what the online platform allows from a technological perspective: Do I have a character limit? Can I embed graphics in my text? Depending on the type of our online exchanges, we may also need to incorporate intonation or emotion to enrich our communication in the form of letter repetition or emoticons. Consequently, different online data may require different methodological approaches for the analysis. The following list presents both social and technological factors that may affect how we communicate online, as well as possible solutions for our analysis.
Word Limit
A lot of online environments allow a specific number of words or characters. SMS used to allow only 160 characters per message; in a similar fashion, Twitter allowed only 140 words per tweet. Users came up with creative ways of communicating using limited word space, such as abbreviations and acronyms. When analyzing data from such sources, we need to make sure to do some “data cleansing” before performing any kind of further analysis: expanding such abbreviations and acronyms will greatly improve the recall and accuracy of our queries. Nowadays, text analysis tools frequently include common online abbreviations and acronyms in their spell check dictionaries.
Typos
Typing on a keyboard very often results in typos: spelling mistakes that occur from mistyping words, either because of the key size or speed –or sometimes both. Using a spell check during the process of data cleaning is always a good idea, but it is mandatory in the case of online communication for better results in our analysis. Text analysis tools have been trained to recognize spelling errors occurring from key proximity and are able to accurately correct them in an automated manner.
Channels/Mode of Communication
With the appearance of Web 2.0, a lot of platforms became increasingly multimodal. Online communication used to be purely textual, which is why users introduced emoticons to better convey emotion in the absence of a richer medium, such as a picture. However, we see more and more pictures or icons embedded in text, which may result in illegible strings or URLs after our data collection. This is particularly true on Twitter, where emojis are used very frequently. Depending on the purpose of our analysis, we may wish to remove such noise or transform it into a form that may be useful for our analysis.
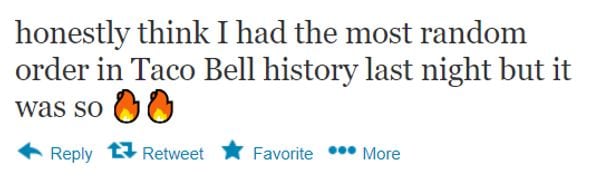
For example, emojis carry important information for sentiment analysis; sometimes, it may be the only element carrying sentiment in a tweet, as in the picture below ( = fire = positive). Instead of disregarding this valuable information, a custom dictionary with emoji codes and their descriptions may be used to replace icons or illegible strings with words that a text analysis tools would recognize.
Metadata
Noise may also be created by metadata, or, in other words, “data about data”. A lot of text in online communication may be accompanied by useful information that usually appears in a structured manner. For example, when collecting data from Twitter, each tweet may have information about the user, the date it was posted, the location, etc. Depending on the tool used for collecting the data, this information could appear in a structured, organized table, or all jumbled together as one single text. In the latter case, we would have to clean up the metadata if we are only interested in the tweet content, or extract the information we need from it and input it in a table.
Quotes
A lot of online platforms allow quoted text to be included in the text of other users. This may also introduce noise in our data that could affect our analysis (for example, identifying sentiment that is quoted by replying to the review of another user). It is usually recommended to identify patterns for quoted text and remove it during the data cleansing stage, before proceeding with further analysis. The following is a review quoting another customer’s review in a forum that allows embedded quoting. During data collection, the quoted text may be collected along with the actual post, which would create issues in sentiment analysis. In this case, we could identify the pattern used for indicating quoted text in our data and remove the quoted review that may already appear in our dataset.
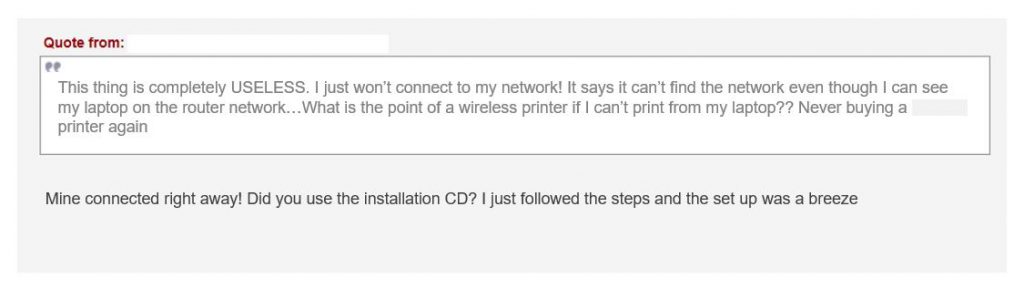
Paralinguistic Aspects
When communicating face-to-face, the tone and pitch of our voice, as well as non-verbal cues such as our facial expression, adds further meaning to out words. Especially in text-only environments, the lack of such features has been compensated for by letter repetition, capitalization, spaces between letters, formatting, emoticons, etc. The use of such structures may affect our recall in queries for specific words; for example, the verb “love” in “loooooove my new phone” would not be a recognizable string for a computer; this is where the addition of spell check dictionaries comes in handy, so that we can transform such strings into recognizable forms by text analysis tools.
Demographic characteristics in data source
A lot of demographic characteristics such as gender and age are an important part of our social identity –and our social identity affects the way we use language. Knowing the user demographics of our data is especially helpful in online communication, where certain phenomena are usually correlated with gender or a certain age group. Different spelling or morphological conventions, as well as vocabulary used for expressing gender and/or age identity may affect the validity of our results. Being aware of such differences is especially helpful in sentiment analysis, where, depending on gender or age, we may find different positive or negative terms, or structural ways of expressing sentiment intensity. The word “die” in the following tweet is usually associated with negative sentiment, but younger generations may be use it to express positive sentiment.
Moreover, the capitalization and the word “literally” adds more emphasis to the positive sentiment, which could be accounted for in a text analysis tool that captures sentiment degree.
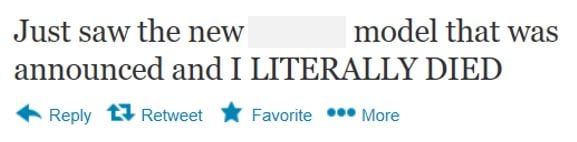
Online Community Membership
A lot of research has been done on the nature of online communities and how it affects the language used by the members of different communities. Being a member of a community, online or offline, is another part of our social identity and we tend to express our membership through the use of linguistic features that are associated with our community. In the absence of physical presence in cyberspace (in the majority of cases), this phenomenon may intensify. As with demographic characteristics, identifying and understanding whether there is a specific online community at the source of our data may improve our entity extraction, sentiment analysis, and social network analysis.
Online Community Norms
Another social factor related to online communities are their social norms: rules that the members have agreed upon and are required to follow, as another expression of their membership. A good example is Tumblr, where the users of certain communities add any personal opinions or comments in the field intended for information retrieval tags instead of the main text4. Unless we are aware of such practices, we may dismiss records as not including sentiment in sentiment analysis, because we may have discarded the text in the tags field as “metadata noise”. For example, one of the tags “I love the colors” is an explicit positive sentiment for the decor of Taco Bell that should not be disregarded as metadata.
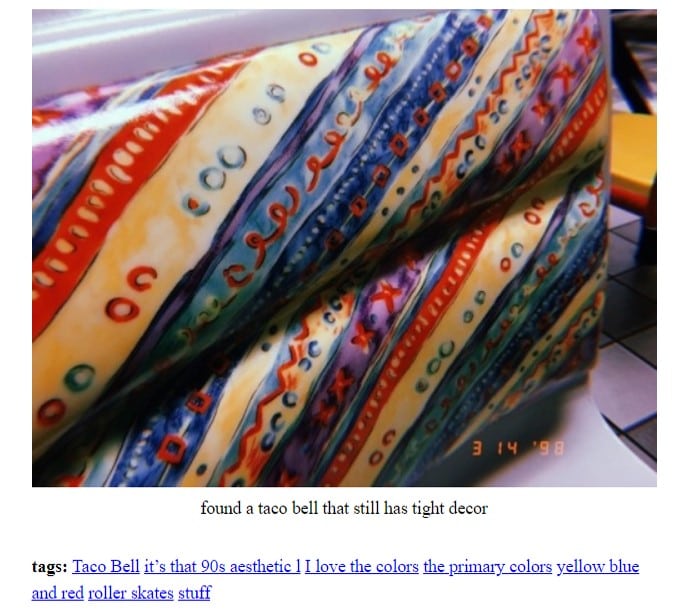
Analyzing online communication with Text Analytics software
We provide machine learning software that can help you make sense of online communication. Using text analytics, you can explore concepts such as consumer sentiment, learn from online product reviews, monitor public opinion on Twitter, stay on top of competitive intelligence, automate the classification of email communications, and so much more.
- Herring, S. C. (1996a). Introduction. In S. C. Herring (Ed.), Computer-mediated communication: Linguistic, social and cross-cultural perspectives (pp. 1-10). Amsterdam: Benjamins.
- https://www.domo.com/learn/data-never-sleeps-5?aid=ogsm072517_1&sf100871281=1
- Herring, S. C. (2007). A faceted classification scheme for computer-mediated discourse. Language@Internet, 4, article 1.
- Bourlai, E. E. (2017). ‘Comments in tags please!’: Tagging practices on Tumblr. Discourse, Context, and Media, doi:10.1016/j.dcm.2017.08.003